In an increasingly connected world, real-time information has become critical to all businesses. To extract, store and analyse heaps of information efficiently, data has to be stored in a scalable manner that allows the business to respond in real-time. Apache Spark and Hadoop were built for precisely this purpose.
Apache Hadoop is an open-source software library that enables reliable, scalable, distributed computing. Apache Spark is a popular open-source cluster computing framework within the Hadoop ecosystem. Both entities are useful in big data processing. They both run-in distributed mode on a cluster.
Hadoop and Spark are two popular open-source technologies making our lives simpler today. They have always been close competitors with their own fan base in the world of data analytics.
Interestingly, both are Apache projects with a unique set of use cases. Though both have a wide array of advantages and disadvantages, they are still pretty easily comparable to decide which one is better for your business.
Let us dive deep and try to understand what these two technologies stand for, through a thorough analysis of their benefits and use cases.
What is Hadoop?
The Apache Hadoop project has been around for a while, but its origins lie in the late 1990s. Doug Cutting and Mike Cafarella created it at Yahoo. Ever since then, it’s become one of the most widely used distributed file systems in the world.
The Hadoop framework is written in Java and enables scalable processing of large data sets across clusters of commodity hardware, leading to high-performance computing. In simple terms, Hadoop is a way to store and process data in an easy, cost-effective way. Hadoop uses a distributed processing model that allows users to access the information they need without storing it on a single machine.
It is used for distributed storage and database management by businesses, governments, and individuals. Hadoop can also be considered a cluster computing platform that uses the MapReduce programming model to process big data sets.
Hadoop was created mainly to address the limitations of traditional relational databases and provide faster processing of large data sets, particularly in the context of web services and internet-scale applications.
The four major modules of Hadoop are:
- Hadoop Distributed File System (HDFS): This system stores and manages large data sets across the clusters. HDFS handles both unstructured and structured data. The storage hardware that is being used can be anything from consumer-grade HDDs to enterprise drives.
- MapReduce: MapReduce is the processing component in the Hadoop ecosystem. The data fragments in the HDFS are assigned to separate map tasks in the cluster. MapReduce processes teh chunks to combine the pieces into the desired result.
- Yet Another Resource Negotiator: YARN is responsible for managing job scheduling and computing resources.
- Hadoop Common: This module is also called Hadoop Core. It consists of all common utilities and libraries that other modules depend on. It acts as a support system for other modules.
What is Spark?
Apache Spark is an open-source project by Databricks and supports the processing of fast data sets in real-time. Databricks provides Spark as a service and now offers more than 100 pre-built applications in different domains. It’s used for interactive queries, machine learning, big data analytics and streaming analytics.
Spark is a fast and easy-to-use in-memory data processing framework. It was developed at UC Berkeley as an extension of the big data ecosystem that has been supported by Hadoop, Apache HBase, Hive, Pig, Presto, Tez, and other components since its inception. Spark engine was created to boost the efficiency of MapReduce without compromising its benefits. Spark uses Resilient Distributed Dataset (RDD), which is the primary user-facing API.
It provides an optimised distributed programming model in which computations are carried out in a distributed manner on clusters of machines connected by high-speed networks. Its technology is specially devised for large-scale data processing. It reduces the task of handling huge amounts of data by breaking it into smaller tasks that can be processed independently.
It also offers a distributed computing framework based on Java for big data processing. Spark uses Scala and Python programming languages, and it is open source.
The Five Major Components of Apache Spark –
- Apache Spark Core: This component is responsible for all the key functions like task dispatching, scheduling, fault recovery, input and output operations, and much more. Apache spark core acts as a base for the whole project, and all functionalities are built on it.
- Spark streaming: As the name suggests, this component enables the processing of live data streams. The live stream data can originate from any of the sources like Kinesis, Kafka, Flume, etc.,
- Spark SQL: In this component, Spark gathers all information about the structured data and processing information of the data structures.
- Machine Learning Library (MLLib): This component consists of a vast library of machine learning algorithms. The goal of a machine learning library is to make it scalable and make machine learning more accessible.
- GraphX: It consists of a set of APIs that can be used for facilitating graph analytics tasks.
Hadoop vs Spark: Key Differences
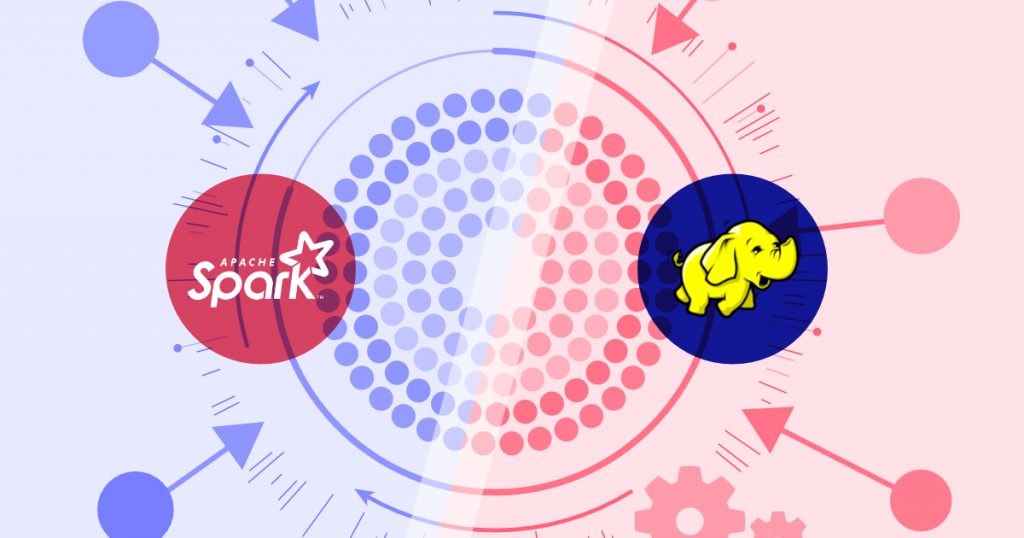
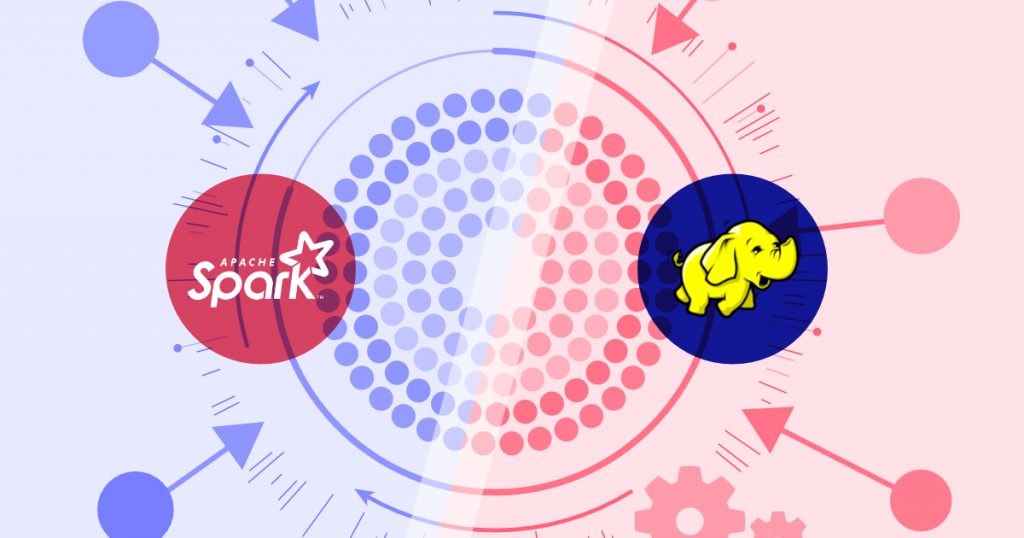
Hadoop is a mature enterprise-grade platform that has been around for quite some time. It provides a complete distributed file system for storing and managing data across clusters of machines. Spark is a relatively newer technology with the primary goal to make working with machine learning models easier.
Apache Hadoop and Apache Spark are the two giants in the big data world. While many of us cannot tell the exact difference between them, understanding them is pretty important. Both have their pros and cons; it all depends upon what you are looking for and what your needs are.
Both are distributed computing solutions and each has value in the right circumstances. Choosing between Hadoop and Spark can be a difficult task, as there is no easy “winner” or black-and-white answer to the question. The best approach for your business will likely depend on what you are currently working with, your team’s skill sets, and your long-term strategy.
Let’s now look into the differences between Hadoop and Spark on different parameters –
Performance
Performance is the most important metric that drives the success of any data analytics software and platform. The performance of Hadoop and Spark has been a major topic of debate since the release of Apache Spark. But how different is the performance one from the other? Is one better than the other? Is it even possible to compare Hadoop and Spark?
Performance comparison between Hadoop and Spark is inevitable. Unfortunately, comparing them based on performance is not as easy as we would like to believe. Several factors contribute to performance in a big data environment, including software choice, hardware capabilities, number of nodes used, storage availability etc.
Hadoop Boosts the overall performance when it comes to accessing the locally stored data on HDFS. But when it comes to in-memory processing, Hadoop can never match with Spark. Apache claims that when using adequate RAM for computing, Spark is 100 times faster than Hadoop through MapReduce.
In 2014, Spark set a new world record in sorting the data on disk. Spark was able to dominate Hadoop by being three times faster and using 10 times fewer nodes to process 100TB data on HDFS.
The main reason for Spark’s high performance is that it doesn’t write or read the intermediate data to the storage disks. It instead uses RAM to store the data. On the other hand, Hadoop stores the data on many different levels. After this, the data is processed in batches using MapReduce.
Spark might seem to be a complete winner here. However, if the size of data is larger than the available RAM, then Hadoop will be the more logical choice.
Cost
A recent article published by IT consultancy firm Frost & Sullivan said that Hadoop continues to generate a positive Return On Investment (ROI) for enterprises. The same firm also predicted that Spark is poised to expand its market share in the enterprise computing space.
Directly comparing the price between these two big data processing frameworks is a very simple task. Since both the platforms are open-source, they are completely free. But the organisation must factor in the infrastructure, development, and maintenance cost to get the Total Cost of Ownership (TCO).
When it comes to hardware, Hadoop works on any type of data storage device for processing data. This makes the hardware cost for Hadoop relatively low. Apache spark, on the other hand, relies on in-memory computation for its real-time data processing. Spark typically requires spinning up plenty of nodes, which, in turn, require lots of RAM. This makes hardware cost for Spark relatively higher.
Finding a resource for application development is the next significant factor. Since Hadoop has been around for a long time now, it is easy to find experienced software developers. This makes the demand slightly lower; hence you have to shell out a lesser remuneration. It’s not the same case with Spark, where it is a tad more difficult to find resources.
It is important to note that even though Hadoop seems to be relatively cost-effective, Spark processes data at a much faster rate, making the ROI almost similar.
Data Processing
Both the frameworks process data in a distributed environment; Hadoop does it with MapReduce, while Spark executes it with RDDs. Both handle data in different ways. But, when it comes to real-time processing, Spark shines out. However, Hadoop is the ideal option for batch processing.
The Hadoop process is pretty simple — it stores the data in a disk and analyses the data in parallel in batches over a distributed system. MapReduce, on the other hand, can handle large amounts of data with minimal RAM. It relies only on hardware storage. Hence it is best suited for linear data processing.
Apache Spark works with RDD. It is a set of elements stored across the clusters in the partition of nodes. The RDD’s size is usually too large for a single node to handle. So Spark partitions RDD in the closest node and performs the operation in parallel. Directed Acyclic Graph (DAG) is used in the system to track all RDD performances.
With high-level APIs and in-memory computation, Spark can handle live streams of unstructured data very effectively. The data is stored in several partitions. A single node can have many partitions, but a single partition cannot be expanded to another node.
Fault Tolerance
Both the frameworks provide a reliable solution to handle failures. The fault-tolerance approaches of both systems are quite different.
Hadoop provides fault tolerance based on its operation. The Hadoop system replicates the same data multiple times across the nodes. When an issue arises, the system resumes work by filling missing blocks from other locations. There is a master node that tracks all the slave nodes. If a slave node doesn’t respond to the master node’s pinging, the master node assigns the remaining job to another slave node.
In Spark, tolerance is handled by RDD blocks. The system can track the creation of an unchangeable dataset. It restarts the process when there is an error in the system. Spark uses DAG tracking of the workflow to rebuild data in clusters. Hence this system enables Spark to handle issues in a distributed data processing system.
Scalability
Hadoop rules this section. Remember, Hadoop uses HDFS to deal with big data. As the data keeps growing, Hadoop can easily accommodate the rising demand. On the other hand, Spark doesn’t have a file system, it has to rely on HDFS when handling large data. This makes it less scalable.
The computing power can be easily expanded and boosted by adding more servers to the network. The number of nodes can reach thousands in both frameworks. There is no theoretical limit on how many servers can be added to clusters and how much data can be processed.
Studies show that in the Spark environment, 8000 machines can work together with petabytes of data. On the other hand, Hadoop clusters can accommodate tens of thousands of machines with data close to exabyte.
Ease of Use and Programming Language Support
Spark supports multiple programming languages like Java, Python, R, and Spark SQL. This makes it more user-friendly and allows developers to choose a programming language that they are comfortable with.
Hadoop’s framework is based on Java, and it supports two main programming languages to write MapReduce code: Java and Python. The user interface of Hadoop is not that interactive to aid users. But it allows developers to integrate with Hive and Pig tools to enable writing complex MapReduce programs.
Apart from its support for APIs in multiple languages, Spark is also very interactive. The Spark-shell can be used to analyse the data interactively with Python or Scala. The shell also provides instant feedback to queries.
Programmers can also reuse existing code in Spark, which reduces application development time for developers. In Spark, the historic and stream data can be combined to make the process more effective.
Security
Hadoop seems to have the upper hand when it comes to security. To make matters worse, the default security feature is set to ‘Off’ in Spark. Spark’s security can be amped up by introducing authentication via event logging or shared cost. However, this is not sufficient for the production workloads.
Hadoop has multiple authentication features. The most difficult one to implement is Kerberos authentication. Other authentication tools that Hadoop supports include Ranger, ACLs, Service level authorisation, inter-node encryption, LDAP, and standard file permission on HDFS. Apache Spark has to integrate with Hadoop to reach an adequate level of security.
Machine Learning
Since machine learning is an iterative process, it works best in in-memory computing. Hence Spark shows more promise in this area.
Hadoop’s MapReduce splits jobs into parallel tasks. This makes it too large for data science machine learning algorithms to handle. This creates an I/O performance issue in Hadoop applications. The main machine learning library Mahout in Hadoop relies on MapReduce to perform classification, clustering, and recommendation.
Spark has a default machine learning library that can perform iterative in-memory computation. Spark also has data science tools to perform classification, pipeline construction, regression, evaluation, persistence, and more.
Spark is the best choice for machine learning. MLlib is nine times faster than Apache Mahout in a Hadoop disk-based environment.
Resource Management and Scheduling
Hadoop uses external solutions for scheduling and resource management as it doesn’t have an inbuilt scheduler. In a Hadoop cluster with node manager and resource manager, YARN is responsible for resource management. Oozie is a tool available for scheduling workflows in Hadoop.
Hadoop MapReduce works with scheduler plugins like Fair Scheduler and Capacity Scheduler. These schedulers make sure that the cluster’s efficiency is maintained with essential resources.
Spark has these functions inbuilt. The operations are divided into stages using DAG Scheduler in Apache Spark. Every stage has multiple tasks to execute which are handled by Spark and DAG scheduler.
Using Hadoop and Spark together
Both the frameworks are unique and come with lots of benefits. These platforms can do wonders when used together. Hadoop is great for data storage, while Spark is great for processing data. Using Hadoop and Spark together is extremely useful for analysing big data. You can store your data in a Hive table, then access it using Apache Spark’s functions and DataFrames. These are the two major components of Apache Spark that enable you to analyse big data in real-time.
The Spark framework is intended to enhance the Hadoop framework and not to replace it. Hadoop developers can boost their processing capabilities by combining Spark with HBase, Hadoop MapReduce, and other frameworks.
Spark can be integrated into any Hadoop framework like Hadoop 1.x or Hadoop 2.0 (YARN). You can integrate Spark irrespective of your administrative privilege to configure the Hadoop cluster. To sum up, there are three ways to deploy Spark in your Hadoop framework: YARN, SIMR, and standalone.
Uses Cases of Hadoop
Hadoop has a wide range of applications, such as market analysis, scientific research, financial services, web search, and e-commerce. Let us look into them –
- Hadoop is the go-to platform for an organisation that requires the processing of large datasets. Typically, it is a befitting choice for any scenario where the data size exceeds the available memory.
- When it comes to handling large data, financial sectors cannot be ignored. The use of data science is very prominent in this sector. They often use large data to analyse and assess risks, create trading algorithms, and build investment models. Hadoop has been a huge help to build and run these models successfully.
- If you are low on budget, then Hadoop is the right and effective framework to choose. It allows you to build data analysis infrastructure on a low budget.
- Retailers often analyse a large set of structured and unstructured data to understand their audience and serve their customers in a much better way. Hadoop is a great go-to tool for these retailers.
- Hadoop can also be used in organisations where time is not a constraint. If you have large datasets to be processed and you don’t want it immediately, then Hadoop could be your choice. For example, eCommerce sites can use Hadoop to boost their engagement rate.
- Suppose your organisation depends on large machinery, telecommunication, or a large fleet of vehicles. In that case, you can send all the big data from the Internet of things (IoT) devices to Hadoop for analysis. Hadoop-powered analytics will make sure all your machinery is scheduled for preventive maintenance at the right time.
Use Cases of Spark
Here are some scenarios and situations where Spark can be used instead of Hadoop –
- If real-time stream data processing and analysis are essential for an organisation, then Apache spark is the go-to option for you.
- Spark is enabled with an in-memory computation feature that allows organisations to get all the results in real-time. So industries in which real-time results make a difference can leverage the Spark framework.
- The iterative algorithm in Spark helps to deal with chains of parallel operations. Organisations that are dealing with multiple parallel operations can use this tool to their benefit.
- There is a library of machine learning algorithms available with Spark. This enables you to use high-level machine learning algorithms in processing and analysing your datasets.
- Apache Spark can be used in health care services. Healthcare providers are using Apache Spark to analyse patients’ records and the past clinic data in real-time.
- The gaming industry has also started utilising Spark. Spark analyses the users in real-time and in various game events to suggest lucrative targeted advertising.
Summary
In this post, we have seen the key difference between Hadoop and Spark. Hadoop typically allows you to process and analyse large data sets that can exceed the drive capacity. It is primarily used for big data analysis. Spark is more of a general-purpose cluster computing framework developed by the creators of Hadoop. Spark enables the fast processing of large datasets, which makes it more suitable for real-time analytics.
In this article, we went over the major differences between Hadoop and Spark, the two leading big data platform of choice. We also laid some ground work for which you should use over another and when.
To conclude, Spark was developed with the intention to use it as a support to boost Hadoop’s functionality. When both frameworks are used together, you can enjoy the dual benefits of both.
Looking for the perfect cloud-driven security for modern enterprises? Cloudlytics has got the perfect solution for you. Contact us now for any queries.