As the popularity of Kubernetes platform for deploying container-based applications keeps soaring every passing day, the challenge of keeping it secure and away from prying eyes has increased manifold. With every container you add, the area prone to attack widens, and so does the number of entry points for malicious activities.
So, if you are scaling without keeping in check the security, you are exposing critical data to unauthorized users and thereby putting your organization at a severe security risk. This is why businesses have started employing Kubernetes audit logs for Kubernetes logging and monitoring. It allows you to mitigate the risk of unwanted access and misconfigurations, along with protecting your organization against abuse of its Kubernetes resources.
This blog discusses everything you need to know about Kubernetes audit logs, including best practices to follow to excel at it.
What are Kubernetes Audit Logs?
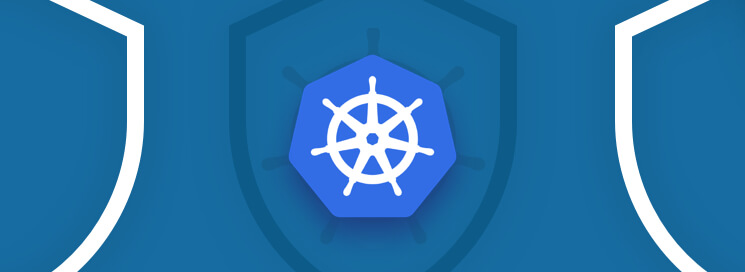
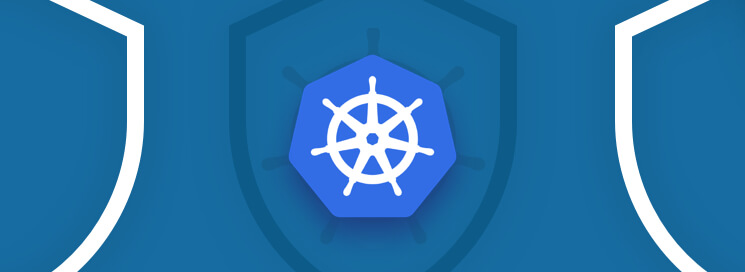
Kubernetes audit logging was first introduced in Kubernetes 1.11. It ensures your Kubernetes remain secure and away from unauthorized access.
Kubernetes API is the centre of everything that happens within Kubernetes. Audit logs are structured in JSON, with each log containing rich metadata. For example, it includes the requested URL path, the HTTP method, and the user information sending the request.
Kubernetes API server processes all the changes to Kubernetes state and stores the same as backend storage. It makes these servers ideal for Kubernetes audit logs to send record requests to. This allows capture of every modification taking place within a cluster. This is because API server processes requests from a wide array of internal and external elements, such as –
- Itself
- The control plane
- Nude daemons (example – the kube-proxy and the kubelet)
- Users making kubectl requests
- Cluster services, such as CoreDNS and kube-state-metrics
- Items sending requests through a kube client, such as applications, controllers, and operators
Why Should You Monitor Kubernetes Audit Logs?
Attackers use a myriad of ways to get access and modify Kubernetes clusters, including resources, services, and accounts. At the same time, most of the techniques rely on benefitting from basic misconfiguration pertaining to your RBAC policies or the Kubernetes environment. So it is imperative to have a Kubernetes audit log analysis to track how a cluster is behaving.
Kubernetes audit logs are turned off by default. The default settings allow users to store Kubernetes events in the backend database for up to one hour. But it can be insufficient when you are looking to track unauthorized access.
Enabling audit logs would allow you to capture events and modify actions (create, update, read, or delete).
It also lets you track the heartbeats sent from a node to the API server. The kube-apiserver is responsible for auditing a Kubernetes cluster. So whenever a request creates a namespace, it is sent over to it for logging and auditing.
It means that by integrating Kubernetes audit logs, you can figure out the answers to the following –
- What happened?
- Who did it?
- When did it happen?
- What was the location of such an occurrence?
Therefore, whenever a malicious activity happens within a cluster, the actions are automatically registered in the audit log. It then brings your security tools into play. They parse the events available in the audit log and inform the requisite parties about such unwanted access and modification.
It is imperative to understand that a Kubernetes cluster is a happening place, i.e., there is a lot of activity going on, but you need not record every single instance of every such occurrence. Having a well-rounded audit policy lets you filter critical events from the rest and record them separately.
How to Monitor Kubernetes Audit Logs?
Depending on your Kubernetes audit policy, the API captures specific information in your audit logs.
It is divided into stages or events –
- RequestReceived – It is generated as soon as the audit handler receives a request. There is no processing involved here.
- ResponseStarted – These are generated after the response headers are sent but before sending the response body.
- ResponseComplete – It is generated after sending the response body.
- Panic – It is generated when panic takes place.
Not every stage applies to all the requests. In addition, you can also define the audit level to inform the system of the level of event that has to be recorded.
We can distinguish audit levels into four primary categories –
- None – The system doesn’t log events containing this string.
- Metadata – It logs request metadata, such as resource and timestamp, but skips recording the request or response bodies.
- Request – It doesn’t apply for non-resource requests and logs event metadata and request body only. It skips the response body.
- RequestResponse – It logs the entire event, including its metadata, request, and response bodies. But this command is inapplicable for non-resource requests.
Given the intricacies involved, it is critical to deduce a policy that filters requests specific to user needs, such as pods, secrets, and kube-proxy.
Here is a sample audit policy –
apiVersion: audit.k8s.io/v1 # This is required.
kind: Policy
# Don't generate audit events for all requests in the RequestReceived stage.
omitStages:
- "RequestReceived"
rules:
# Log pod changes at RequestResponse level
- level: RequestResponse
resources:
- group:" "
# Resource "pods" doesn't match requests to any subresource of pods,
# which is consistent with the RBAC policy.
resources: ["pods"]
# Only check access to resource "pods"
- level: Metadata
resources:
- group:" "
resources: ["pods/log", "pods/status"]
# Don't log watch requests by the "system:kube-proxy" on endpoints or services
- level: None
users: ["system:kube-proxy"]
verbs: ["watch"]
resources:
- group:" "# core API group
resources: ["endpoints", "services"]
# Don't log authenticated requests to certain non-resource URL paths.
- level: None
userGroups: ["system:authenticated"]
nonResourceURLs:
- "/api*" # Wildcard matching.
- "/version"
# Log the request body of configmap changes in kube-system.
- level: Request
resources:
- group:" "# core API group
resources: ["configmaps"]
# This rule only applies to resources in the "kube-system" namespace.
# The empty string "" can be used to select non-namespaced resources.
namespaces: ["kube-system"]
# Log configmap and secret changes in all other namespaces at the Metadata level.
- level: Metadata
resources:
- group:" "# core API group
resources: ["secrets", "configmaps"]
# A catch-all rule to log all other requests at the Metadata level.
- level: Metadata
# Long-running requests like watches that fall under this rule will not
# generate an audit event in RequestReceived.
omitStages:
- "RequestReceived"
Kubernetes allows you to place multiple audit rules in a Kubernetes audit policy, but when the system processes an event and compares it to them, it is only the first matching rule that sets the event’s audit levels. It is a far cry from RBAC and Kubernetes policy, where the most restrictive rule is the one that leads the charts.
How Do You Enable Audit Logging in Kubernetes?
While there are multiple audit rules, there are specific fields for defining each one of them –
- level – It defines the range or the verbosity of the event.
- resources – The object to be audited.
- nonResourcesURL – It defines a virtual location not associated with any other resources.
- namespace – It defines objects within a namespace subject to audit.
- verb – It defines a specific operation for the current audit, such as create or delete.
- users – It is the authenticated user for the current rule.
- userGroups – It is the authenticated user group for the current rule.
- omitStages – It allocates stages where the system can skip generating events at.
Since the Kubernetes audit logs are turned off by default, you will have to execute a function to activate it. One way of doing it is passing the audit-policy-file flag during the process of initiation of kube-apiserver in addition to the audit policy defined by you.
The last step is to specify the path to the file using the audit-log-path function. It would provide a location to the API. If you do not do it, it will output logs to Stdout by default in JSON format.
Challenges involved
While establishing Kubernetes audit logs seems an easy task to master, it is far from it. Even though the principles of audit logs collection and analysis apply to the cloud, and more specifically, to the data centres based on Kubernetes automatically, the dynamic nature of the environment makes audit logs an expensive, cumbersome, and tedious task.
Given that a cluster houses thousands of daily log entries, it is only sane not solely to depend on manual inspection to chalk out irregularities. Even the quietest clusters with no human intervention regarding actions and no shift in applicative activity process many API calls every hour. These are generated by the internal Kubernetes mechanisms that work to ensure that the cluster is alive and functioning and its resources are utilized according to the established deployments. In addition, it helps identify and recover from anomalies and failures automatically.
So, even using a log filtering tool doesn’t mean you have your task cut out. You will require proactiveness, experience, and intuition to figure out minute yet sensitive entries that often pass unabated.
The dynamic nature of Kubernetes also adds to its audit log challenges. You will find multiple workloads added, removed, and modified every second. It means you will require a keen eye and an in-depth understanding of sensitive databases to figure out anomalies. Also, there can be instances when an activity has previously not been recorded by the system. In such cases, it would be dicey to depend on alerts altogether. Instead, you will have to employ additional tools to understand the context and move accordingly.
Kubernetes Audit Logs Best Practices
By now, it is clear that Kubernetes comes with its set of challenges, and those can be hard to tackle unless you have a set pattern. Thankfully, we have you covered. Here are the Kubernetes audit logs best practices which should act as a reference for you –
1) Establish a Logging system
While it is not difficult to activate and reach Kubernetes audit logs, the real challenge is to choose a location to make them easily accessible, even in an emergency. It is imperative to have a dedicated and centralized access point to ensure these remain separated from the rest of your data.
2) Try Avoiding Sidecars for the Logging System
One of the best Kubernetes audit logs practices you can follow is to have a single container instance per pod. It allows you to have the same image for multiple instances of the same container stored in the same pod. In addition, it enables you to have the same container replicated within the pod based on the same instance.
Having a sidecar means creating an additional container for capturing the output of the first container that houses your actual app. It not only takes up extra space but also slows down your system’s processing speed as it has more data to now fiddle with. But it can also be unavoidable in circumstances where you do not have control of the app or have the container away from the logging framework.
In such cases, you can establish a system to collect all logs from the entire node having a single container or not collecting anything otherwise.
3) Writing Logs to Stdout and Stderr
While it is a norm to shift to a containerized environment, we have come across a host of companies focusing on apps that log to files. Allowing logs to redirect to Stdout and Stderr enables you to have a centralized logging framework that can then stream the logs to your desired location more constructively. It also lets you have more control over the data being distributed and place additional filters for better bifurcation.
4) Setting Resource Limits on Log Collection Daemons
Kubernetes logs are difficult to manage at the cluster level, given the large volume. But DaemonSets enables you to run containers in the background and deploy similar containers with pods meeting specific criteria. You can use different software to collect logs in Kubernetes and run them with your workloads using DaemonSets. But for optimizing your log file collection endeavor, you need to set a resource limit per daemon.
5) Having a Robust Retention Mechanism
Audit logs eat a lot of space, and your system’s memory is in red before you even realize it. So it is necessary to have a clear retention policy devoid of any ambiguity that pans out the road ahead for storing and referencing audit logs. Therefore, if you are going for a higher retention policy, you will have to chalk out the probable cost associated with it. Several vendors offer managed logging services that help curtail your infrastructure budget, but security is often a factor deterring people from choosing them.
6) Use SIEM Tools for Improved Alerts
By default, Kubernetes allows users to generate audit logs on API-based invocations. But you must tackle unwanted behavior by enabling the Kubernetes logging and monitoring facility to ensure optimum security. In addition, you can also track illegal changes in your clusters by leveraging operational Kubernetes monitoring logs.
These additional layers inform you of anomalous behavior and help track vulnerabilities leading to potential cyberattacks. It is where SIEM tools come into play. These let you raise alerts automatically and set up a robust and customized dashboard for optimum visualization and generating improved and actionable Kubernetes security data.
7) Distinguishing Between Production and Development Clusters
When you do not have distinguished clusters for development and production purposes, you often end up deleting crucial data too soon or preserving stale information for longer than required. This is why you should have different production and development clusters. It would also mean having different storage locations for both. Kubernetes allows its users to switch seamlessly between the two by using the kubectl config use-context command.
How Cloudlytics Helps Monitor Kubernetes Audit Logs?
By now, you may have already understood that Kubernetes audit logs are a complex exercise. It allows you deeper visibility into cluster operations, helps you develop a robust container infrastructure and enables you to track anomalies. But given the dynamic nature of the Kubernetes environment, it is imperative to keep a tab of additional features and improvements.
At Cloudlytics, we provide clients real-time visibility into their cloud applications and infrastructure. We provide a SaaS solution helping you garner insights for your cloud security. In addition, we simplify the complexities involved in the Kubernetes audit logs and help optimize the compliance of your business operations with set standards.
Click here to explore our services.